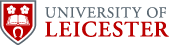
This session invites presentations dealing with structured metadata in a standardized form across the survey life-cycle: models, systems and tools for i.e. instrument design, data entry, data processing, maintaining data documentation, and capturing and storing the metadata within a repository for later reuse. There is increased interest in supporting the comparison and harmonization of studies/waves over space and time, and across studies, especially at the level of theoretical concepts, questions, and variables to which structured metadata is well suited.
Capturing metadata as early on in the survey life-cycle as possible in a structured way enhances transparency and quality, enables reproducible research and reuse of survey components for other waves or surveys.
A wide range of different products and services for different users can be generated on the basis of computer-processable metadata like web-based information systems, traditional codebooks, command setups for statistical packages, question banks, and searching and locating of data which assist in the use or interpretation of the data.
Papers are invited on, but not limited to, the following topics: reuse of metadata across space, time, and studies, metadata banks such as for questions and classifications, metadata-driven processes, and metadata-driven information systems, possibly using the major specification for social science metadata, DDI Lifecycle (DDI 3 branch of the Data Documentation Initiative).
The session is aimed at survey designers and implementers, data and metadata managers, information system managers of cross-national surveys, metadata experts, and others.
Session at the 9th International Conference on Social Science Methodology Research Committee on Logic and Methodology RC33
Conference dates: 11-16 September 2016
Venue: University of Leicester, UK
Deadline: 21 January 2016